The NHS needs to take back control of its data
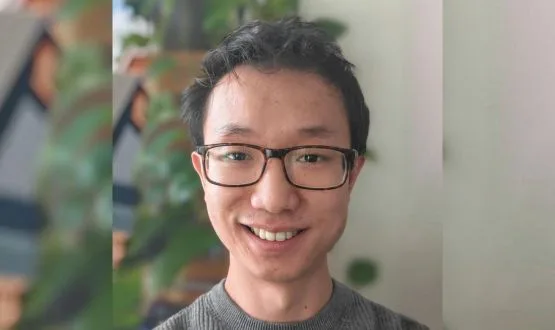
By Dr Joe Zhang, Head of Data Science, Artificial Intelligence Centre for Value Based Healthcare and London Secure Data Environment
Robust data infrastructure and language AI could transform the NHS, addressing the challenges of unstructured data and the critical need for ethical, transparent data governance in healthcare.
The NHS sits on a goldmine of clinical data, but much of its value remains locked away behind clinical records software, or in unstructured form – buried within clinical notes, letters, and free-text fields. The data we have available nationally are a poor quality and shallow representation of patients, poorly suited for AI or detailed research.
While most medical journeys are recorded electronically, the suppliers that create clinical software often do allow analysts and researchers to access the data that is generated. To provide access, suppliers will often charge substantial amounts for the privilege.
In addition, more than 80% of information is recorded in free text. While some companies have demonstrated the value of manually extracting insights from clinical notes, this approach isn’t scalable across a health system as vast as the NHS. The good news is that we now have the technology to automate this process through language AI, potentially unlocking unprecedented value from NHS data.
This isn’t just about operational efficiency; it’s about fundamentally improving patient care and research capabilities. By using improving access to deeper healthcare data, and properly utilising language AI at scale, we can accelerate patient access to clinical trials, enhance real-world evidence generation for life sciences research, and – perhaps most critically – address systematic biases in our healthcare data.
The hidden bias in healthcare data
One of the most compelling arguments for leveraging language AI lies in its potential to address healthcare inequalities. Consider a typical GP consultation: when seeing a patient with multiple comorbidities from a deprived area, perhaps struggling with English, time constraints may mean only the most pressing conditions get properly coded. In contrast, simpler consultations allow time for comprehensive coding. The result? Patients with complex needs may end up with sparser coded records, creating a dangerous data bias that can perpetuate healthcare inequalities.
This bias isn’t just a documentation issue – it has far-reaching implications for AI development in healthcare. When we train AI models on structured data alone, we risk embedding these biases into our algorithms. By incorporating unstructured data through language AI, we can build more complete patient profiles and develop more equitable AI solutions.
What we can learn from other countries
Looking beyond our borders, we can learn valuable lessons from other countries and industries. Singapore, Switzerland and Scandinavian countries, have shown remarkable success in healthcare data management, largely due to their focus on system harmonisation and standardised EHR/EPR implementations. But perhaps the most important lesson comes from non-healthcare industries: the emphasis should be on infrastructure and data pipelines rather than algorithm development.
Healthcare has fallen into what I call the “algorithm fallacy” – developing countless algorithms on clean, curated datasets that fail to perform in real-world conditions. In contrast, successful industries build robust data pipelines and infrastructure, regularly retraining their models to adapt to changing real-world conditions. This is especially crucial in healthcare, where patient conditions and care pathways are inherently dynamic.
The value proposition: an uncomfortable but necessary conversation
We need to have frank, open discussions about the value of NHS data. Currently, hospital data is being sold to private companies who clean it, extract value, and broker it onwards – all while the NHS sees little return on this valuable asset. This fragmented approach not only fails to maximise the potential of our data but also raises serious concerns about data governance and patient trust.
The value chain needs to be brought back into the NHS. While this might be an uncomfortable conversation, it’s essential for ensuring that any monetary value generated from NHS data directly benefits patient care and system improvement. By controlling and properly managing our data assets with continual patient engagement and maximum transparency, we can ensure both ethical use and financial sustainability.
Infrastructure before innovation
The path forward requires a fundamental shift in our approach. Rather than focusing solely on developing new algorithms, we need to invest in robust data infrastructure and pipelines. This means creating systems that can handle real-world data complexity, support regular model retraining, and ensure consistent data quality across the system. This also mean changing procurement practices and legislation, such that the NHS (not the systems supplier) is always in control of its data.
This infrastructure-first approach would enable us to achieve several critical objectives. By building robust data pipelines, we could extract deeper data, and more meaningful insights from unstructured data at scale, transforming millions of clinical notes into actionable intelligence. Such infrastructure would also allow us to maintain up-to-date, representative AI models that evolve with our patient population and clinical practices. It would ensure equitable representation in our healthcare data, addressing the current biases that risk perpetuating health inequalities. Perhaps most importantly, this approach would create sustainable value from NHS data assets, ensuring that the benefits of data-driven healthcare flow back into improving patient care and system efficiency.
At the AI Centre, we’re actively working to address the architectural challenges, developing scalable solutions for handling unstructured data and building the robust data pipelines necessary for sustainable AI deployment. Our focus in the London Secure Data Environment isn’t on creating more algorithms, but rather on establishing the foundational infrastructure that will allow existing AI solutions to work effectively in real-world clinical settings.
The road ahead
Success requires more than just technical solutions though. We need recognition of the challenge, appropriate funding, and strategic focus. Equally important is the governance framework that ensures public acceptability and ethical use of data.
As we move forward, we must maintain transparency in our objectives and be honest about both the challenges and opportunities. The NHS has a unique opportunity to lead in ethical, effective healthcare data utilisation, but this requires careful navigation of technical, cultural and governance challenges.
Dr Zhang will take part in a Rewired 2025 session ‘NHS data architecture: where we are, where we need to get to’ exploring the size of the potential prize in securing data trust with the public and working with the wider life sciences community. He will be joined by speakers Ele Harwich, director at Newmarket Strategy and Will Browne, co-founder of Emrys Health.